Pixel-accurate Semantic Segmentation of Aerial Images with fully convolutional neural Nets
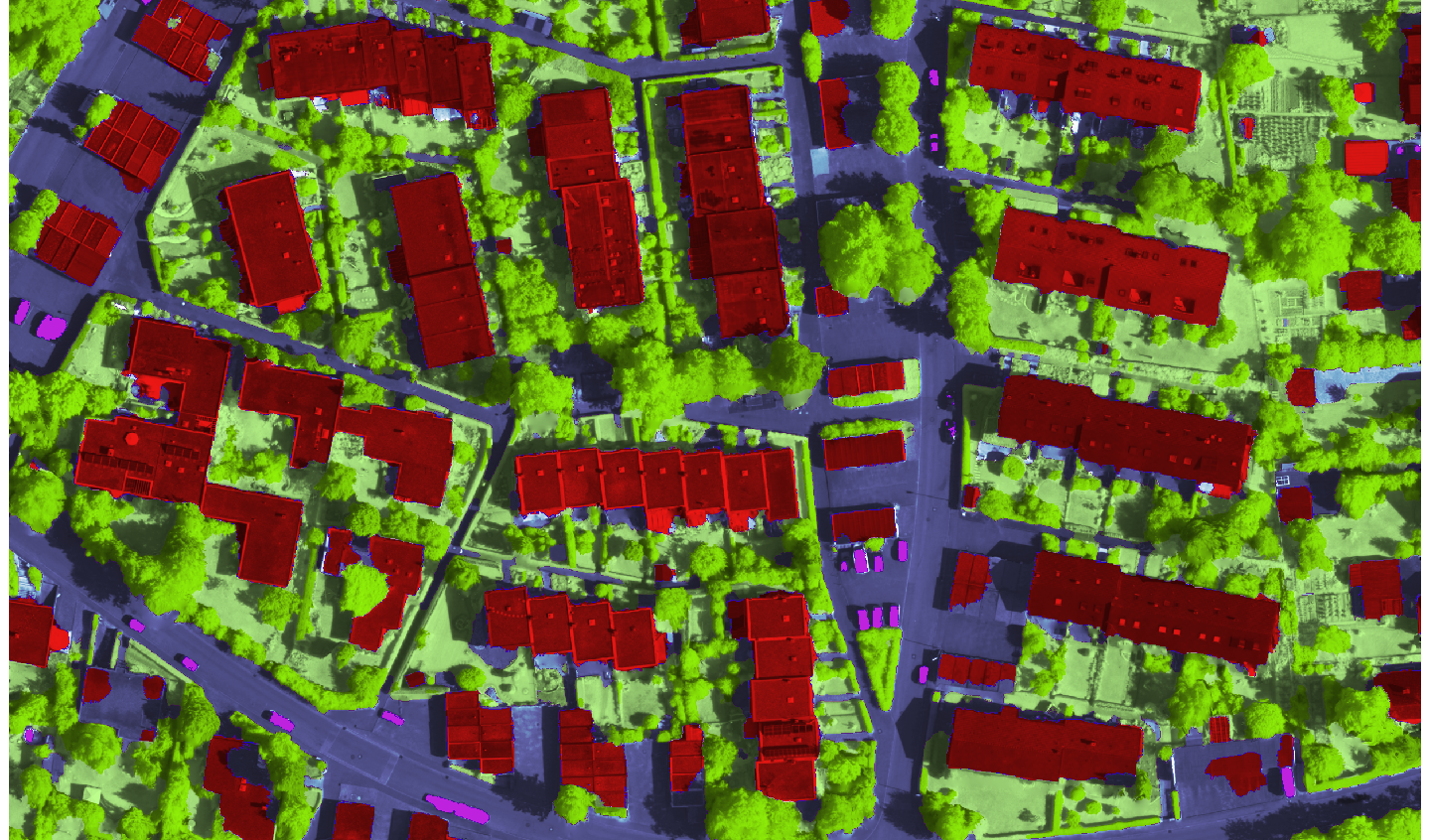
Large amounts of very high resolution (VHR) remote sensing images are acquired daily with either airborne or spaceborne platforms, mainly as base data for mapping and earth observation. Despite decades of research the degree of automation for map generation and updating still remains low. In practice, most maps are still drawn manually, with varying degree of support from semi-automated tools. What makes automation particularly challenging for VHR images is that on the one hand their spectral resolution is inherently lower, on the other hand small objects and small-scale surface texture become visible. Together, this leads to high within-class variability of the image intensities, and at the same time low inter-class differences. We are developing deep learning methods to annotate images such that each pixel receives a particular class label that associates it to a category like "building", "trees" or "impervious surface". Semantic segmentation of aerial images is an important step to interpret their content. It can also be viewed as a prerequisite for many applications like rapid hazard response actions after natural disasters, automatic map generation, or navigation.
Representative Publications:
Marmanis, D., Wegner, J.D., Galliani, S., Schindler, K., Datcu, M., Stilla, U.: Semantic segmentation of aerial images with an ensemble of fully convolutional neural networks, ISPRS Annals of the Photogrammetry, Remote Sensing and Spatial Information Sciences, 2016.
Marmanis, D., Kerr, Grégoire H. G., Wegner, Jan D., Schindler, Konrad, Datcu, Mihai, Stilla, Uwe: Learning Class-Contours of Very High Spatial Resolution Aerial Images Using Convolutional Neural Networks (PDF, 6.4 MB), ODAS-2016, 16th Onera-DLR Aerospace Symposium, Oberpfaffenhofen, 2016.
Contact person:
Jan Dirk Wegner and Dimitris Marmanis (DLR/TUM)